Artificial Intelligence (AI) has emerged as a transformative tool in many industries, and the midstream oil and gas sector is no exception. In an industry that has historically relied on complex, high-cost operations, AI presents an opportunity to optimise processes, improve efficiency, and enhance decision-making.
AI technologies like machine learning, data analytics, and automation can help midstream oil and gas companies address some of their most pressing challenges.
By leveraging AI, companies can analyse vast amounts of data from drilling operations, production equipment, and even environmental conditions to make more informed decisions faster than ever before.
With AI's ability to forecast trends, companies can make more strategic decisions that reduce unnecessary spending, improve resource allocation, and cut down on expensive manual processes.
AI can process and analyse large datasets in real time, providing valuable insights that help decision-makers understand complex situations and make data-driven choices with confidence. AI can monitor real-time data to detect potential hazards, enabling better safety protocols and minimizing risks to personnel and equipment.
By adopting AI, the midstream oil and gas industry is not just improving operations; it's paving the way for a smarter, more sustainable future. Whether it's optimising exploration, production, or refining, AI can be the key to unlocking more efficient and cost-effective operations across the entire value chain.
#AI Applications in Oil and Gas
AI technologies are increasingly being applied in the oil and gas industry to solve complex challenges, streamline operations, and improve decision-making.
Here are some specific AI applications that are transforming the industry:
Predictive Analytics
Predictive analytics uses AI algorithms and machine learning models to analyse historical data and predict future events or outcomes. In midstream oil and gas, this application is particularly useful in predicting equipment failures, production rates, and market trends.
Maintenance and Equipment Monitoring: AI-powered predictive maintenance systems can monitor equipment performance in real-time. By analysing sensor data from pumps, turbines, and other machinery, AI can predict when a component is likely to fail, allowing for proactive maintenance and reducing unplanned downtime.
Production Optimisation: Predictive analytics can also forecast optimal production rates based on data from previous drilling operations, allowing companies to adjust operations and increase output while minimising waste.
Demand Forecasting
This is crucial for the midstream oil and gas industry to predict market trends and adjust production accordingly. AI helps companies make more accurate predictions by processing vast amounts of historical market data, consumer behavior, and geopolitical factors.
Price Prediction: Machine learning models can analyse historical price trends, supply chain disruptions, and geopolitical events to forecast oil prices with a higher degree of accuracy.
Consumption Patterns: AI can also predict future energy demand by analysing variables like seasonality, weather patterns, and industrial activity. This helps companies align their production with market needs, avoiding both overproduction and shortages.
Risk Management
AI applications in risk management help midstream oil and gas companies identify, assess, and mitigate potential risks across operations.
Operational Risk Analysis: AI models can analyse historical data to identify patterns and trends that indicate potential operational risks, such as pipeline failures, equipment breakdowns, or accidents.
Safety and Environmental Risks: AI-driven tools can monitor environmental conditions in real-time, such as changes in weather, seismic activity, or equipment stress levels, to predict safety hazards or environmental risks.
Financial Risk Management: AI models can also analyse financial data, market conditions, and regulatory changes to assess risks to profitability, compliance, and long-term financial health.
Real-Time Data Analysis
Real-time data analysis enables oil and gas companies to make quick decisions based on the most current data available, enhancing operational efficiency and safety.
Drilling Operations: During drilling operations, real-time data from sensors, drilling equipment, and geological surveys can be analysed by AI to optimise drilling parameters, such as speed and pressure.
Production Monitoring: AI algorithms can monitor production parameters such as flow rates, temperature, and pressure in real-time. This data can be used to identify inefficiencies, detect anomalies, and optimise production operations.
Supply Chain and Logistics: AI can also be used to manage and optimise supply chains, ensuring timely delivery of resources to production sites and refining facilities. By analysing data from multiple sources, AI can predict delays and suggest alternatives to minimise disruptions.
#Impact of AI on Exploration and Production
AI is revolutionising the oil and gas industry by enhancing exploration, production, and reservoir management.
Let’s explore how AI is impacting these critical areas:
Enhancing Exploration with AI
Exploration is one of the most resource-intensive and time-consuming stages in the midstream oil and gas industry. Traditional exploration methods rely on seismic surveys, geological mapping, and drilling to discover new reserves. AI improves this process by analysing geological data and identifying potential drilling sites more efficiently.
Seismic surveys generate massive amounts of data that geophysicists must analyse to identify potential oil or gas reservoirs. AI-powered algorithms can process this data faster and more accurately, detecting patterns and anomalies that may indicate the presence of oil or gas.
AI can integrate geological, geophysical, and petrophysical data from different sources to create more accurate subsurface models. These integrated models help geologists and exploration teams make more informed decisions about where to drill, saving both time and money.
AI models can analyse historical exploration data to identify successful drilling patterns and reduce exploration risks. This data-driven approach can guide exploration teams toward areas with the highest probability of success, significantly improving the return on investment for new exploration projects.
Improving Drilling Accuracy with AI
Drilling is another crucial part of oil and gas production, and even small inaccuracies can lead to costly mistakes, including wellbore instability, equipment failure, or lost production. AI enhances drilling operations in several ways to improve precision, minimise errors, and optimise performance.
AI systems can analyse real-time data from sensors embedded in drilling equipment to optimise drilling parameters. Machine learning algorithms can adjust drilling speed, pressure, and other factors based on this data, reducing the risk of wellbore instability and other issues.
AI-driven autonomous drilling systems can automatically adjust drilling operations to maintain optimal conditions without human intervention. These systems help improve drilling accuracy by minimizing human error and maximizing efficiency.
AI algorithms can analyse equipment health and usage patterns to predict when a component might fail. This helps in planning maintenance and repairs before breakdowns occur, reducing downtime and ensuring that drilling operations continue smoothly.
Optimising Production with AI
Maintaining optimal production levels is crucial for maximising output and profitability. AI plays a significant role in improving production by continuously analysing real-time data from wells and production facilities.
Machine learning algorithms identify patterns in this data and recommend adjustments to equipment settings or operational strategies that can increase production efficiency and reduce resource waste.
AI can monitor production systems for abnormal conditions, such as leaks, equipment malfunctions, or other inefficiencies, and alert operators in real-time. Early detection of issues allows quicker intervention and minimises the potential for costly delays or environmental hazards.
AI systems can forecast production rates over time based on historical data, well performance, and reservoir conditions. This helps companies better manage their production schedules, optimise resource allocation, and plan for future operational needs.
Enhancing Reservoir Management with AI
Efficient reservoir management is essential for maximising recovery and extending a reservoir's life. AI’s ability to process and analyse large volumes of data from sensors, production logs, and other sources provides insights that can significantly improve reservoir performance.
AI-powered models can simulate reservoir behavior by analysing historical data. These simulations help engineers understand how a reservoir will respond to different extraction methods, enabling more accurate planning of well placement and production techniques.
AI can optimise enhanced oil recovery (EOR) techniques, such as injecting fluids (water, gas, or chemicals) to increase production from mature fields. Machine learning models can predict the best EOR techniques based on reservoir characteristics and past performance, leading to more effective recovery and higher yields.
By detecting subtle changes in reservoir parameters, AI can identify issues that might impact long-term production, such as reservoir depletion or the onset of reservoir damage. This proactive monitoring allows for timely interventions, preserving reservoir health and maximising recovery.
#Challenges and Opportunities of AI
The widespread adoption of AI also faces several challenges that companies must address. Conversely, the sector has significant opportunities to capitalise on AI’s future development and innovation.
Let's delve into the challenges and the opportunities AI brings to the midstream oil and gas industry.
One of the biggest challenges in implementing AI within the midstream oil and gas sector is ensuring the quality and availability of data. AI systems rely on high-quality, accurate data to learn, train, and make predictions. Data is often unstructured, siloed across various departments, or incomplete in the energy industry. These silos make it difficult to create unified, comprehensive datasets that AI can analyse effectively.
Inconsistent or noisy data, especially from legacy equipment or outdated sensors, can lead to inaccurate AI models and predictions.
AI technologies require skilled personnel to implement, monitor, and maintain them. The midstream oil and gas sector faces a shortage of professionals with the necessary technical expertise in AI and an understanding of the industry’s specific challenges and processes.
There is a growing demand for data scientists, AI engineers, and other skilled professionals. However, there aren’t enough qualified professionals with the required expertise in AI and oil and gas operations, which can slow down the pace of adoption.
Existing employees may require significant training to adapt to AI tools and systems. This includes familiarising them with new technologies and encouraging a data-driven mindset across the workforce.
Despite the challenges, AI offers tremendous opportunities for growth and innovation in the midstream oil and gas sector. As AI technologies evolve, several promising areas for the energy industry can benefit.
AI is poised to drive automation to new levels, reducing human intervention in risky or repetitive tasks, optimising resource allocation, and improving the speed of decision-making. This can lead to significant cost savings and productivity improvements.
AI’s ability to predict when equipment will fail is one of the most exciting opportunities for the oil and gas sector. Predictive maintenance powered by AI can reduce unplanned downtime, minimise repair costs, and extend the lifespan of equipment.
AI technologies can enhance safety in the midstream oil and gas industry by improving risk detection and response times. Real-time data analysis can identify hazards before they escalate into accidents, ensuring the safety of workers and reducing environmental risks.
AI can help midstream oil and gas companies improve their environmental performance by optimising energy usage, reducing waste, and minimising emissions. AI-powered systems can model the environmental impact of various operations and recommend more sustainable practices.
#Real-World Case Studies of AI in Oil and Gas
Oil and gas companies are increasingly adopting AI to improve efficiency, optimise operations, and reduce costs. Here are a few case studies where AI has been successfully implemented in the industry, along with the outcomes and benefits:
1. Shell: Predictive Maintenance and Production Optimisation
Overview: Shell, one of the world's largest oil and gas companies, has been at the forefront of integrating AI into its operations. One of their notable projects involved using AI for predictive maintenance and optimising production processes.
AI Application: Shell implemented AI-driven predictive maintenance to monitor its offshore platforms and drilling rigs. The AI system analyses sensor data from equipment like pumps, turbines, and compressors to predict when these machines will likely fail. Machine learning algorithms identify patterns in the data, flagging potential issues before they lead to equipment breakdowns.
Outcomes and Benefits:
Reduced Downtime: Predictive maintenance significantly reduced unplanned downtime by enabling Shell to perform maintenance before equipment failures occurred. This resulted in higher operational availability.
Cost Savings: By predicting and preventing failures, Shell saved money on emergency repairs and avoided costly production interruptions.
Increased Production Efficiency: The ability to detect faults early allowed Shell to optimise maintenance schedules, which improved production efficiency and extended the lifespan of critical equipment.
2. Total: AI in Gas Leak Detection
Overview: Total, a major multinational oil and gas company, used AI to enhance safety and environmental protection on its offshore platforms by improving gas leak detection.
AI Application: Total deployed AI-based gas leak detection systems use machine learning algorithms to analyse data from sensors monitoring the environment. These systems can identify anomalies in real time and predict potential gas leaks before they occur. The AI system is trained to recognise patterns associated with different types of gas leaks and their severity.
Outcomes and Benefits:
Early Leak Detection: The AI system enabled early detection of potential gas leaks, reducing the risk of environmental damage and ensuring the safety of workers on offshore platforms.
Improved Safety: By identifying gas leaks before they become hazardous, the system was able to reduce the risk of explosions and other safety incidents, protecting both the environment and workers.
Operational Efficiency: AI-driven monitoring helped streamline safety checks and reduced the need for manual inspections, saving both time and operational costs.
These case studies illustrate the wide-ranging benefits of AI in the oil and gas sector, from improving operational efficiency and reducing costs to enhancing safety and sustainability.
As AI continues to evolve, its potential to transform the midstream oil and gas industry will only grow, leading to even more innovative solutions and greater operational improvements.
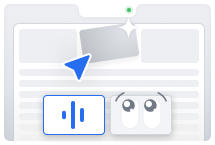