#Introduction to Predictive Maintenance
In the midstream oil and gas sector, equipment reliability is crucial. Downtime, even briefly, can lead to significant operational and financial setbacks. Traditionally, companies have relied on reactive maintenance (fixing equipment after it breaks) or preventive maintenance (scheduled maintenance at regular intervals).
While these approaches are widely used, they have limitations: reactive strategies can lead to unexpected breakdowns, and preventive methods may result in unnecessary servicing and higher maintenance costs.
Enter predictive maintenance (PdM) - a smarter, data-driven approach. Predictive maintenance uses real-time and historical data from equipment to predict when a failure might occur, allowing maintenance teams to intervene just in time. This minimises unplanned downtime, extends asset life and reduces overall maintenance costs.
Thanks to advancements in machine learning (ML) and data analytics, predictive maintenance has evolved rapidly. Machine learning models can process vast amounts of sensor data, detect patterns and make highly accurate predictions about equipment health.
For midstream oil and gas companies, machine learning is transforming maintenance from a cost center into a strategic asset.
#Machine Learning in Maintenance
At the heart of predictive maintenance lies machine learning (ML), a branch of artificial intelligence that enables systems to learn from data and improve their predictions over time.
In the context of midstream oil and gas operations, ML is a game-changer. Equipment like compressors, pipelines, and pumps are often equipped with IoT sensors that collect continuous data on parameters such as temperature, vibration, pressure, flow rates, and more. This data is the fuel for predictive insights.
So, how does machine learning fit in?
Machine learning algorithms analyse this sensor data to detect patterns and anomalies that indicate early signs of wear, malfunction, or potential failure. Here’s how it typically works:
Data Collection: Sensors attached to equipment gather real-time data, sometimes thousands of data points per second.
Data Processing & Feature Extraction: Raw data is cleaned and processed. Key features or signals (e.g., sudden temperature spikes or changes in vibration frequency) are extracted for analysis.
Model Training: Historical maintenance data (such as logs of past failures or repairs) is used to train machine learning models. These models learn to associate certain patterns with specific types of failures.
Prediction & Alerts: Once trained, the ML model continuously monitors incoming sensor data and compares it to learned patterns. When it detects behavior that suggests a failure might occur, it triggers an alert, giving maintenance teams time to act.
Continuous Learning: As more data is collected, the model refines its predictions, improving accuracy and reducing false positives over time.
For midstream oil and gas companies, the application of machine learning in maintenance translates into fewer unexpected shutdowns, optimised maintenance schedules, safer operations and lower operational costs. It's not just about avoiding failures: it's about creating a proactive and intelligent maintenance culture.
#Applications in Midstream Operations
Midstream oil and gas companies manage complex, high-value infrastructure that operates continuously over long distances and in harsh environments. The stakes are high: failures can cause environmental damage, safety hazards, and millions in losses.
That’s why predictive maintenance powered by machine learning is gaining traction in areas such as:
Pipeline Integrity Monitoring
Pipelines are the backbone of midstream oil and gas operations, and ensuring their structural integrity is critical.
Sensors embedded along the pipeline collect data on pressure fluctuations, temperature, flow rates, and even acoustic signals that might indicate a leak or corrosion.
Machine learning models can detect subtle, early-stage anomalies that human operators might miss. For example:
Unsupervised algorithms identify unusual pressure drops that could suggest a pinhole leak.
Time-series models forecast corrosion progression based on environmental and operational conditions.
Early detection helps prevent spills and ruptures, ensures regulatory compliance and reduces costly emergency repairs.
Compressor Maintenance
Compressors are essential for moving gas through pipelines but are prone to wear due to high operating pressures and temperatures.
Vibration analysis, oil temperature, rotational speed, and pressure sensors provide rich data for analysis.
Classification models identify faulty bearings or misalignments by analysing vibration patterns. Predictive models estimate remaining useful life (RUL), enabling just-in-time maintenance.
Anomaly detection models flag deviations in compressor efficiency, which could signal internal component degradation.
This reduces unplanned shutdowns, extends equipment life and optimises repair schedules.
Valve Condition Monitoring
Valves play a key role in regulating flow and pressure. Malfunctioning valves can lead to flow disruptions, pressure build-up, or safety risks.
Sensors track valve position, torque, actuator pressure and response time.
ML models detect sticking, leaking, or slow-closing valves by comparing current behavior to historical patterns. Sequence learning models (like LSTMs) can monitor actuation cycles to detect degradation over time.
This improves operational safety, reduces leakage and ensures that flow control systems operate reliably.
#Benefits for Midstream Companies
Implementing machine learning in predictive maintenance isn't just a tech upgrade, it’s a strategic move that brings substantial operational, financial and safety advantages.
For midstream oil and gas companies, the benefits are as follows:
Reduced Unplanned Downtime
Unscheduled equipment failures are among the most costly events in midstream oil and gas operations.
ML-driven predictive models identify issues before they escalate, allowing maintenance teams to act during planned downtime.
This significantly reduces disruption to operations, maintaining product flow and customer commitments.
For compressors, pumps, or valves, this can mean the difference between a quick fix and a full-blown emergency shutdown.
Extended Asset Lifespan
Equipment in midstream networks is expensive and often operates in harsh conditions.
By identifying subtle degradation patterns early, ML enables timely interventions that prevent further wear.
This extends the usable life of key assets like pipelines, turbines,and control valves.
It also optimises component usage, no more replacing parts as just in case.
Lower Maintenance Costs
Traditional preventive maintenance can be inefficient, either too frequent or too late.
Machine learning allows for maintenance when it's actually needed, cutting unnecessary inspections and part replacements.
It also reduces labor hours and minimises emergency repair expenses, which are often much higher than planned maintenance.
Over time, this leads to a leaner, more cost-effective maintenance program.
Improved Safety and Compliance
Midstream oil and gas operations are heavily regulated, and failures can pose serious health, safety and environmental risks.
ML helps detect risks before they become hazards, such as leaks, pressure anomalies, or mechanical failures.
This proactive approach enhances employee safety, environmental protection, and regulatory compliance.
It also supports better incident reporting and documentation through data-driven insights.
For midstream oil and gas companies, predictive maintenance powered by machine learning is more than just an operational tool: it's a competitive edge.
#Real-World Examples
Here are some case studies where machine learning–based predictive maintenance (PdM) has been successfully implemented in midstream oil and gas operations:
Enbridge – Pipeline Leak Detection and Integrity Monitoring
Enbridge, one of North America's largest pipeline operators, handles thousands of kilometers of oil and gas pipelines.
ML Application:
Enbridge implemented machine learning models for pipeline integrity monitoring and leak detection.
These models analyse real-time sensor data (pressure, temperature, and acoustic signals) to detect anomalies that may indicate corrosion, cracks or leaks.
By training on historical failure data and normal operating conditions, the system reduces false alarms and improves early warning accuracy.
Outcome:
Enhanced early detection of anomalies.
Reduced environmental risks from undetected leaks.
Improved regulatory compliance through reliable monitoring systems.
Shell Midstream – Smart Predictive Maintenance Program:
As part of its digital transformation, Shell Midstream launched initiatives to apply advanced analytics and ML across its pipeline and terminal operations.
ML Application:
Leveraged ML models to predict equipment failures in pumps and motors at storage terminals and pipelines.
Integrated PdM into an enterprise-level asset management platform for automated alerting and action recommendations.
Used natural language processing (NLP) to scan maintenance logs and correlate past failures with sensor data patterns.
Outcome:
Faster root cause analysis of recurring failures.
Improved mean time between failures (MTBF) across several asset classes.
Better alignment between field operations and asset reliability teams.
Kinder Morgan – Compressor Station Optimisation
Kinder Morgan operates a vast network of natural gas pipelines, including hundreds of compressor stations across the U.S.
ML Application:
They partnered with industrial analytics firms to deploy predictive analytics and machine learning models for compressor health monitoring.
Algorithms analysed vibration, pressure and thermal data to predict bearing wear and component fatigue.
Unsupervised ML models flagged anomalies in performance that were previously undetectable with traditional SCADA systems.
Outcome:
30–40% reduction in unscheduled compressor failures.
Significant savings in repair costs and fewer emergency service calls.
Optimised maintenance scheduling, which reduces downtime.
As the midstream oil and gas sector continues to digitalise, the integration of advanced ML techniques will further improve the reliability, safety and sustainability of the energy infrastructure.
Continued investment in data quality, infrastructure and skilled talent will be key to fully realising the potential of predictive maintenance powered by machine learning.
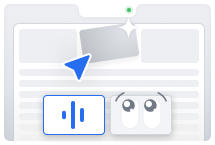